DALL·E 2: what happens when machines make art?

What is DALL·E 2?
DALL·E 2 is an AI created by OpenAI which produces original images in response to a text description. DALL·E 2 can also make edits to existing images (which OpenAI describes as in-painting) and create different variations based on an existing image.
Throughout this article you will find examples of images generated by DALL·E 2 along with the descriptions used to create them.
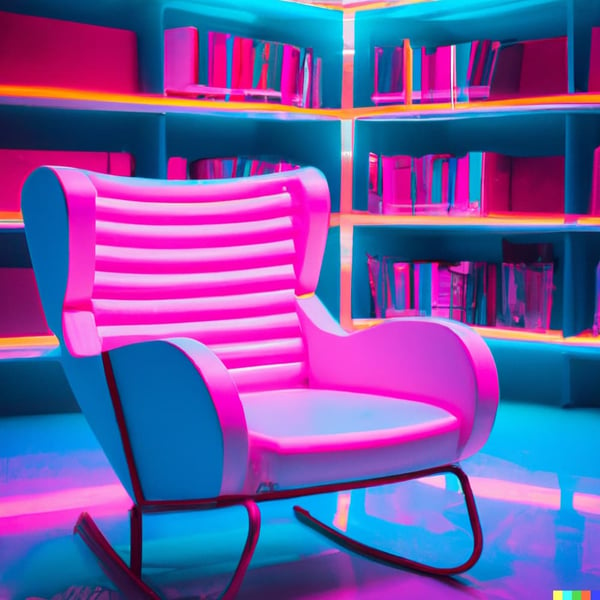
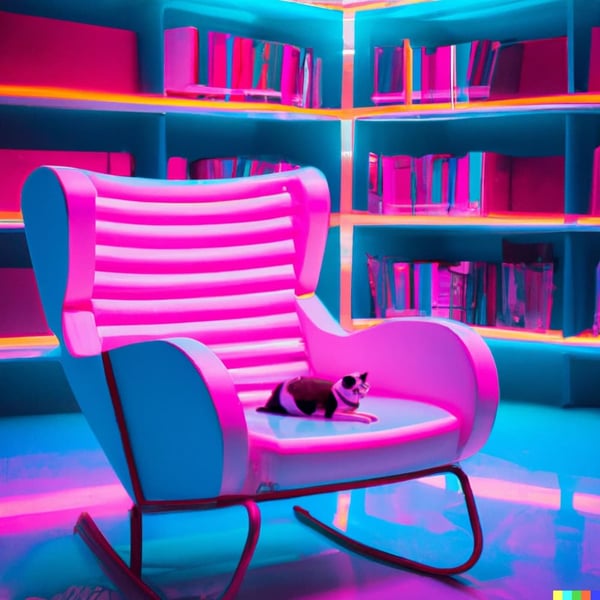
OpenAI recently added an out-painting feature, this allows an existing image to be expanded by adding new content around its edges.
How does DALL·E 2 work?
Under the hood, DALL·E 2 is a series of machine learning models which have been trained to perform the operations required to generate a new image, the first of which is CLIP. Developed by OpenAI and able to encode images and descriptions, CLIP can quantify how well-matched text and image encodings are in a process called contrastive learning. DALL·E 2 uses another model called a prior to select a relevant image encoding for a given text encoding of a description, this image encoding is used to create a gist of the image which will be generated.
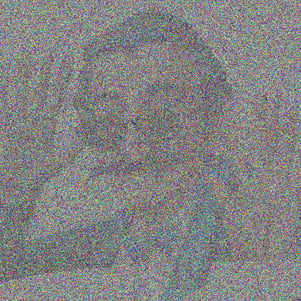
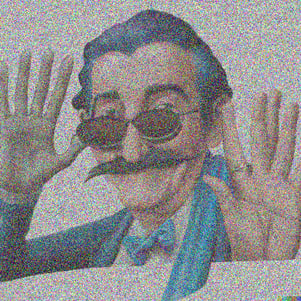
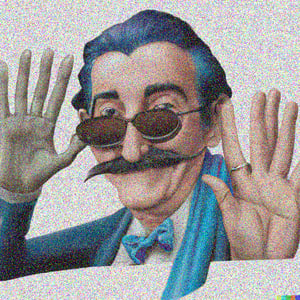
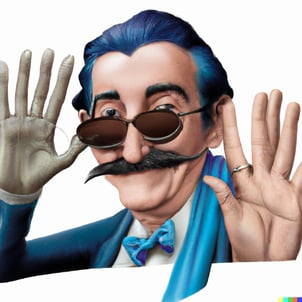
The gist is then passed to a diffusion model. Diffusion models are trained to remove noise from images, but in this case DALL·E 2 uses diffusion to take the gist image and gradually add more detail to it until it is complete, resulting in the generation of an original image corresponding to the entered description.
For a more in-depth explanation of how DALL·E 2 works, please see this article by Aditya Ramesh, the creator of DALL·E and one of the co-creators of DALL·E 2.
What are the potential applications for DALL·E 2?
OpenAI permits the use of images generated by DALL·E 2 commercially, which opens up a world of potential business applications for this technology. It is tempting to imagine that DALL·E 2 can be used anywhere an original image is needed but it has several major limitations (see below) which significantly narrows the field of potential applications.
Replacing stock image libraries may be a potential application, especially given the lower cost of accessing DALL·E 2 when compared to licensing images and the similarities between querying libraries of stock photos and composing DALL·E 2 descriptions. Generating assets for games also seems like a good candidate. Marketplaces like the
e Unity asset store currently offer a variety of assets for use in game development and they can range in price dramatically, DALL·E 2 can be used to quickly and cheaply create some types of assets without requiring the application of artistic skill.
What are the limitations of DALL·E 2?
DALL·E 2 does not have a good understanding of composition. Consider the following example: when asked to create an image containing a series of different coloured shapes, DALL·E 2 succeeds in creating an image with the correct shapes and colours, however; it fails to assign the correct colours to each shape consistently.
This is as a result of how contrastive learning works: CLIP learns which features are sufficient for matching an image with the correct description from the training data it is provided, unless that data includes counter examples (in this case images with captions describing their shape, colour, and position in a variety of combinations), CLIP disregards information about composition.
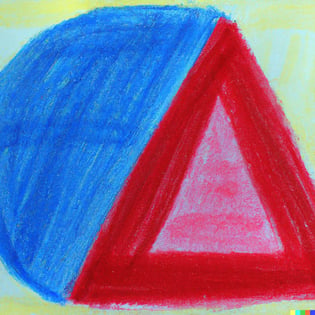
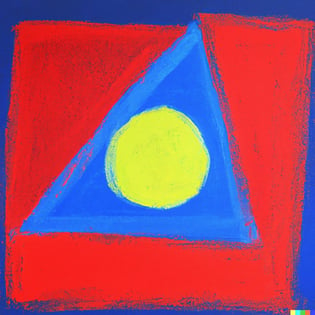
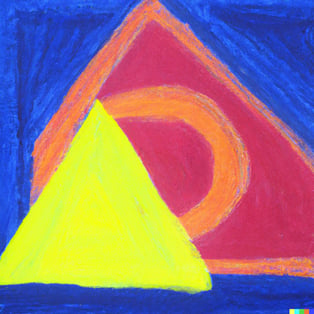
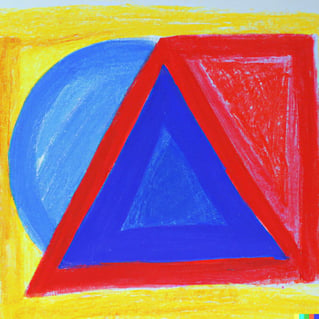
Struggles with composition also prevent DALL·E 2 from being able to recreate coherent text. Given that DALL·E 2 is an image generation AI, and not a specialised text generation AI such as GPT-3 (also created by OpenAI), it is unsurprising that it struggles with text generation.
It is clear that DALL·E 2 understands the concept of text: images containing text often have readable lettering and the text itself often reflects information contained in the description, however; DALL·E 2 struggles with rules on grammar and syntax.
In generated images, words will usually be in the wrong order, and sometimes text generated will be gibberish, especially if the description doesn’t specify the exact wording, resulting in some text that doesn’t feel quite right.
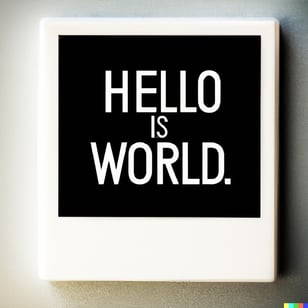
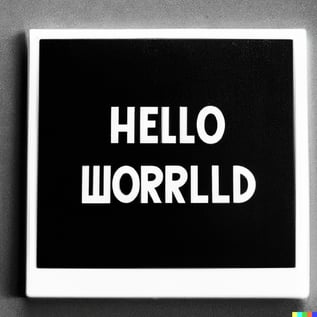
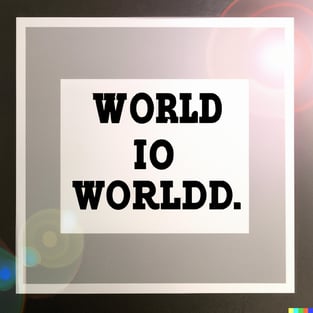
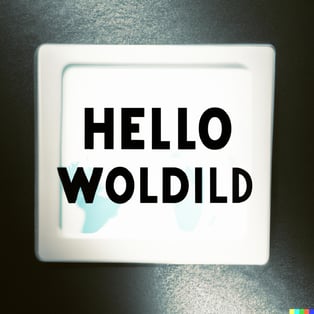
In an effort to prevent DALL·E 2 being used for harmful purposes OpenAI filters text prompts such that DALL·E 2 will not generate images for prompts containing language which is violent, adult, or political. Whilst it is important to prevent the use of image generation AI for harmful purposes these text filters have been known to be overly restrictive at times, with some users reporting seemingly innocent words or phrases being filtered.
In addition to filtering text prompts, OpenAI also uses “advanced techniques” to prevent DALL·E 2 from creating realistic images of real people (including celebrities and public figures). For example, you cannot upload a photo of yourself and modify it with DALL·E 2, at least for now.
Will DALL·E 2 replace human artists in the near future?
Definitely not. DALL·E 2 has some hard limits that ultimately prevent it from competing with human artists. Other image generation AI such as Craiyon (formerly DALL·E mini), Midjourney, and Dream also show promise, but all such systems suffer from similar limitations to DALL·E 2. Image generation AI are based on machine learning models and so are ultimately reliant on the creativity of the artists, photographers, and other creatives who contribute images to the data used to train these models.
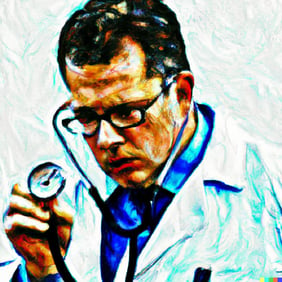
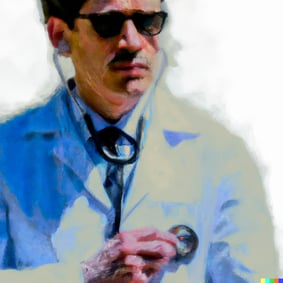
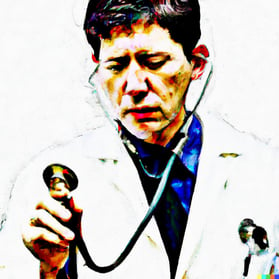
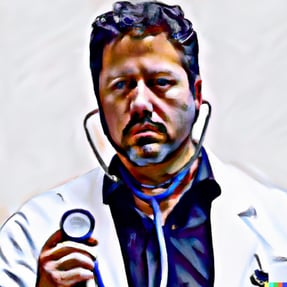
AI are also reliant on the people who label training data. To function accurately and without bias, machine learning models require data that is labelled accurately, consistently, and responsibly. Whilst OpenAI have attempted to address bias in DALL·E 2 the effects of bias are still evident in generated images, for example, descriptions including phrases like “doctor” typically resulting in images of men.
What does the future hold for DALL·E 2?
To answer this question, it can be helpful to look at an example of an older image generation AI technology. GANs (Generative Adversarial Networks) are a class of image generation models trained by using a model that spots AI-generated images to train image generation AI to make better images.
GANs can create original images like DALL·E but only of a specific subject. An example implementation of GANs such as This Person Does Not Exist are effective only because it focuses on a particular type of image — in this case, faces. Despite the limitations associated with using GANs, they have seen wide use and study; rather than replacing human creativity GANs have become a tool used for creative expression.
There is already a dedicated online community experimenting with DALL·E 2 (which has produced some great resources on how to get the best results when writing descriptions) so it seems likely that DALL·E 2 will, like GANs, become a tool used to support human creativity, not replace it.
It is possible to imagine then that 10 years from now, we may see tools such as DALL·E 2 reach a level of sophistication such that using them is more like working in partnership with another person rather than simply using a tool.
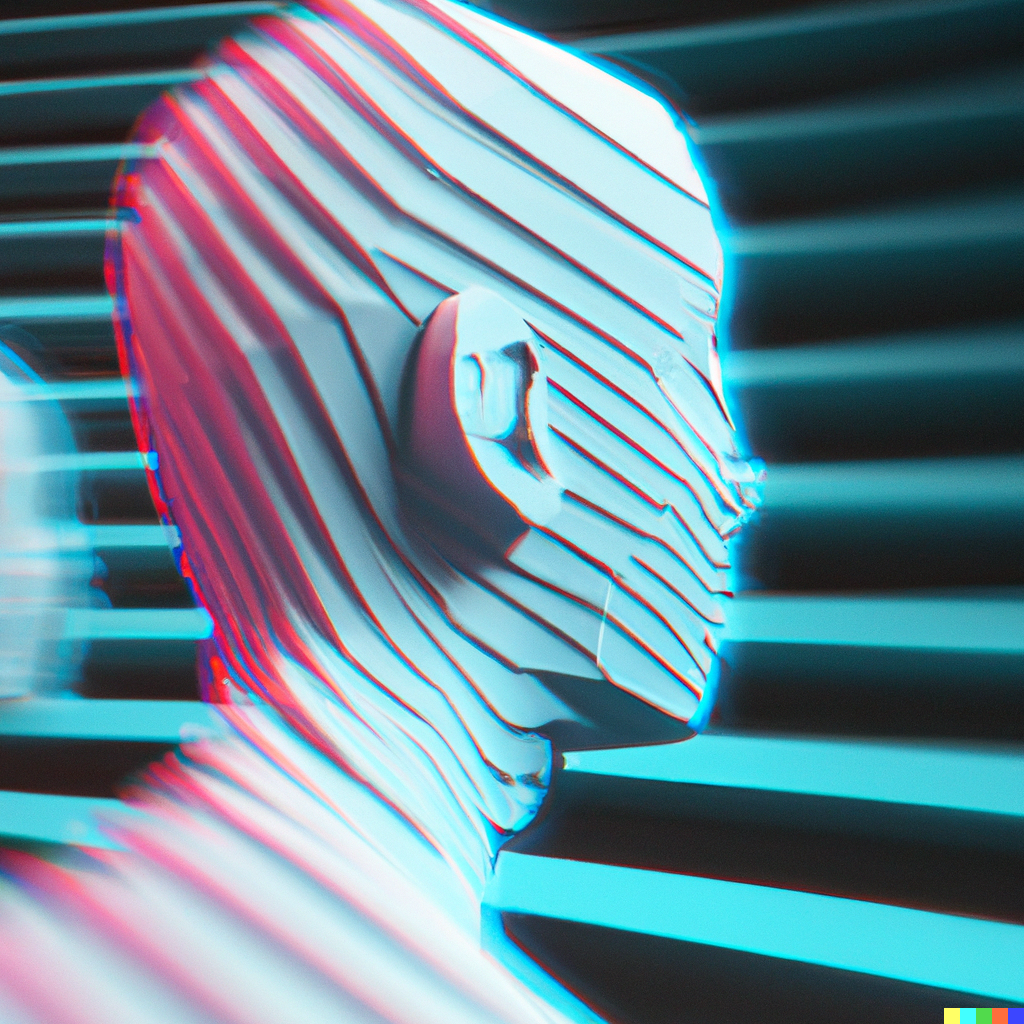
Thanks to Marc Peiser